Page 2 – News Debris For The Week of July 30th
AI Tech is Our Fate.
I’ll start this page with a tutorial. “The Plain English Guide to Machine Learning vs. Deep Learning.”
The business world often uses the terms machine learning, deep learning, and artificial intelligence as interchangeable buzzwords. The problem? Each is uniquely different from its siblings. With so much terminology describing different pieces of the same AI puzzle, it’s easy to misunderstand various components.
Now that you have your bearings, I should point out that Microsoft has been fairly blunt about the risks of AI. “Microsoft warns investors about A.I. risks, including ‘biased information’ and ‘ethical issues’.” The article has highlighted, in a grey box, Microsoft’s concerns. This is only natural. A publicly held corporation must tell its investors about potential risks.
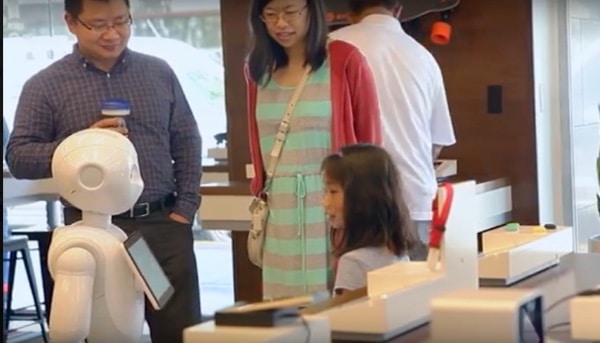
And so as we think about AIs, eventually instantiated in robots or androids, it’s helpful to be aware of related research that may give us pause.
- Could you be emotionally blackmailed by a cute robot?
- New study finds it’s harder to turn off a robot when it’s begging for its life
These two articles suggest that everything we’ve been worried about is coming to fruition. It’s almost as if the AI industry has its own momentum, a fateful drive towards everything we’ve been warning ourselves about. And yet. We forge on.
Perhaps it’s a cultural thing. See: “Why Westerners Fear Robots And The Japanese Do Not.”
In any case, here’s one light and the end of the AI tunnel. “The future role of AI in fact checking.” Author Cousins writes:
As an analyst, I’d like to have a universal fact checker. Something like the carbon monoxide detectors on each level of my home. Something that would sound an alarm when there’s danger of intellectual asphyxiation from choking on the baloney put forward by certain sales people, news organizations, governments, and educators, for example.
But then, that supposes we have great faith on the AI fact checker’s design integrity and lack of bias. Can that be done?
It’s all something to ponder.
Particle Debris is a generally a mix of John Martellaro’s observations and opinions about a standout event or article of the week (preamble on page one) followed on page two by a discussion of articles that didn’t make the TMO headlines, the technical news debris. The column is published most every Friday except for holiday weeks.
John:
A lot to process here, as I’m sure you realise, so let’s dive in.
Your lede, Apple hitting the trillion dollar milestone, what it means and how Apple got there are all interesting, and worthy of study. No doubt, Apple will continue to be studied by business schools and academia in marketing, product design, engineering, human and machine/AI interface, robotics, and military strategy (yes, that too) for generations to come. And while the trillion dollar milestone is as arbitrary as its namesake (milestones in a metric system?), its achievement is no less indelible on our collective psyche, and rightly, commands attention, study and understanding across disciples. Bajarin and Cramer both provide structure for the layman to begin that process. Another time, perhaps.
The issues around AI are, in my view, far more relevant to the lives of the average citizen of any country. Fischer’s piece on ‘Machine Learning vs Deep Learning’ provides not only the basic grammar, but the conceptual structure for understanding what AI is doing. As deep learning is a subset of machine learning, and is a conduit to pattern recognition – the basis by which human beings recognise and categorise both physical and conceptual constructs in fractions of a second, and thereby devote valuable if not life-saving time, energy and resources on appropriate responses with all of the fitness advantages that this confers, we can focus for a moment on machine learning. (NB: pattern recognition underlies the interaction not only between all living systems, but the molecular world including our innate immune systems, the binding of receptors and molecules that form the building blocks of life, but also non-living systems at the atomic and quantum mechanical levels; thus any algorithm that leads to pattern recognition exploits one of Nature’s most universal principles and essential survival tools).
Fischer’s explanation of the fundamentals of machine learning anticipates many of the threats the article on Microsoft identify not simply for their brand, but some of the inherent limitations and ethical challenges inherent in those fundamentals. One is puzzled, however, that neither article identifies one of the system’s greatest inherent weaknesses, namely its dependence on input, and how that shapes algorithmic outcome; specifically if that input is limited by, for example, demographics, then both its generalisable and precise responsiveness and appropriateness are also limited. Fischer cites Netflix as a client that uses AI to suggest programming to their customers. I cannot tell you how seldom Netflix gets it right for my preferences, and it’s not simply about the limits of Netflix’s offerings; as an atypical consumer, they miss me by a wide margin. Generalisable and targeted responsiveness are both at issue here. A more representative user base is required for AI to be more responsive to individual demand.
Apart from whom is engaging AI, how we engage AI is also relevant, as Joi Ito’s ‘Wired’ article on ‘Why Westerners fear robots (and by extension AI) and Japanese do not’ attempts to address. Ito’s argument about cultural differences between Japanese Shinto vs Western Judaeo-Christian constructs is plausible, and likely at least partially explanatory about our relationship to AI/robotics, however it’s the argument about slavery at scale in the West, and its impact on dehumanisation that likely deserves the most attention. Even beyond slavery, it’s our assignment of low social status to the service sector in general that is a legacy of our relationship to serfdom, slavery and 19th/early 20th Century mass labour that is at issue, and how we have achieved a comfort level with its abusive nature by dehumanising the people subjected to these institutions. These have been brutal systems, whose unhappy inductees have struggled to overcome, oftentimes violently; and, Ito argues, it begs the question if our ‘intelligent machines’ will do likewise, possibly to our detriment?
Without debating the merits of this argument, it should be self-evident that treating other human beings as we would have ourselves treated has collateral benefits that extend to how we treat all aspects of our lives, including our resources, devices or AI/robotics. In other words, this is fundamentally a human problem rather than one uniquely confined to the human/AI-robotic interface. Applying this Golden Rule common to all the world’s great religions remains a goal we have yet to achieve, but its benefits to human-human and other relationships will be driven by it.
Finally, Greg Veloria’s take on ‘AI in Fact checking’ strikes me as yet another example of seeking answers to the wrong question. True, the issue is not, or at least should not, be about a database of ‘facts’, but what constitute the relevant facts. This should really not be up to AI. Rather, the true spark of human genius begins with asking the right question, formulating a testable hypothesis and then, through consultation between different minds each with its unique perspective, agreeing upon the relevant facts in a manner that requires a transparent and defensible selection of those facts.
Neither transparency nor defensibility are features now, or perhaps ever, of how AI makes its choices. And that, more than anything else, should drive our choice of whom selects both the question and its relevant facts.
With all this money Apple should reduce the price of its products. They are losing market share on laptops and desktops because of price and no expandability.
I am sure that Apple is doing ongoing analysis and set their price points to bring the best financial return.
All of this fear mongering about AI reminds me what the upper class in previous centuries feared about public education teaching the lower classes how to read.
That was when the Skynet seed was planted. 😀